Vital Signs Technical Appendix: Estimating the Potential Reductions in Clostridium difficile Infection (CDI) among Patients when Antibiotic Use is Improved
To accomplish our third objective, estimating the potential reductions in Clostridium difficile infection (CDI) among patients when antibiotic use is improved, we conducted an observational study of antibiotic exposure and CDI. We also estimated antibiotic usage in a sample of 323 US hospitals from the MarketScan® Hospital Drug Database. Finally, estimates from both the cohort study and antibiotic usage study were used in a stochastic model to estimate the potential reductions in CDI when reducing the usage of high-risk antibiotics. Detailed methods for each analysis are described below.
Observational study of antibiotic exposure and Clostridium difficile infection
We conducted a retrospective study of the relative risk of CDI in 2 large academic medical centers located in New York and Connecticut. The academic center in NY has approximately 700 beds and 40,000 discharges per year while the academic center in Connecticut has approximately 1,000 beds and 58,000 discharges per year. The two academic centers along with their respective State Health Departments are part of the Emerging Infections Program (EIP). The EIP is a network between CDC, State Health Departments and their academic medical center partners to help answer critical questions on emerging infectious threats. Surveillance of CDI is a component of the EIP.
METHODS:
For the study, patients were included if they met the following criteria: 1) 18 years of age or older, 2) had an inpatient stay at a study hospital, and 3) resident of the catchment area included in the Emerging Infections Program (EIP) CDI surveillance.
Data Sources
For the project, we utilized data from the following data sources:
- Hospital Discharge Data: We utilized hospital discharge data from January 1, 2011 through June 30, 2012 to identify a cohort of hospitalized patients. Each participating institution obtained from their hospital discharge database clinical and demographic information such as ICD9-CM procedure and diagnostic codes, patient’s age, sex, date of hospital admission and discharge, inpatient unit(s) visited, disposition at discharge, and initial diagnosis on admission. Patient identifiers were obtained to allow for merging of data with pharmacy and EIP surveillance data.
- Pharmacy Data: Data on antibiotic class, name, route, and duration for all antibiotics administered during each hospital stay was obtained for the same time period of the hospital discharge data. Antibiotics were grouped into the following classes: aminoglycosides, 1st and 2nd generation cephalosporins, 3rd and 4th generation cephalosporins, clindamycin, fluoroquinolones, macrolides, vancomycin, sulfa, beta-lactam/beta-lactamase inhibitor combinations, carbapenems, and miscellaneous. Pharmacy data also contained individual patient identifiers to allow merging of pharmacy data with hospital administrative data.
- EIP Data: The EIP CDI surveillance is an active population and laboratory-based surveillance that has been in place in New Haven County, CT and Monroe County, NY since 2010. It provides longitudinal data on CDI in the residents of the surveillance catchment area. The data collected through the EIP CDI surveillance are entered into a web-based system developed by CDC on a weekly basis and CDC personnel have real time access to the any data entered into the system by the EIP site. We utilized EIP data from the beginning of the surveillance in each site until December 2012 to identify patients diagnosed with CDI in community and healthcare settings.
Data Linkage and Transfer to CDC
Each participating site was responsible for generating a linkable patient identification (LPI) code for the hospital discharge data and the pharmacy data. Encounters identified for the same patient received the same LPI code. The LPI codes were unique to a specific patient, not a specific discharge or pharmacy record, and as such there were multiple discharge or pharmacy records with the same LPI code. LPI codes were linkable to the EIP data.
After the hospital discharge and pharmacy datasets were cleaned and reformatted and the LPI codes added, all other patient identifiers were deleted. Each site also created a CDI database based on the EIP data containing only those patients who developed CDI and who appeared in the discharge/pharmacy data, regardless of the timing of CDI onset relative to the hospital admission. CDI data transferred to CDC did not contain any personal identifiers. CDC was responsible for merging the three datasets, discharge data, pharmacy data, and EIP CDI data, using the LPI codes included in each of the three data sources. The protocol was approved by the institutional review board at each site and CDC.
Analytical Plan
After merging the datasets at CDC, the distribution of all variables was examined, including demographics, frequency and risk of CDI following hospitalization, time from hospitalization to CDI, length of stay (LOS), antibiotic exposure during stay, ICD9-CM diagnosis and procedure categories, inpatient units, and disposition on discharge. For each hospital stay, antimicrobials were grouped into twelve classes, as described above, by the participating sites.
The cohort was created by identifying the first hospital stay within a single year for each unique patient at each of the participating sites, July 2011 to June 2012 for NY and January 2011 to December 2011 for CT. This first stay was defined as the index hospitalization for each patient. Exposures were based on information contained in the hospital administrative data while the outcome was based on the linked EIP surveillance data. Exposures were based primarily on the index hospitalization and subsequent hospital stays up to 180 days. The outcome was defined by development of incident CDI (i.e., positive C. difficile specimen by either toxin or molecular assay in a patient without a positive C. difficile stool in the previous 8 weeks) during either of the following: ≥ 3 days after index hospitalization admission, or within 180 days from discharge from the index hospitalization. In sensitivity analyses, the number of follow-up days post-discharge was varied to 42 days and 0 days instead of 180 days. Patients with CDI in the previous 180 days before the index visit, who died during the follow-up period before development of CDI, or who received any oral vancomycin treatment before documentation of CDI were excluded from the cohort. Additional index hospital visits for the same patient were included if they occurred at least 180 days from the last hospital visit.
We compared patients exposed to certain antibiotics considered to pose a high-risk for subsequent development of CDI during their index hospitalization and subsequent hospitalizations, to patients without exposure to antibiotics. Specifically, the antibiotics classes considered to be high-risk were 3rd/4th generation cephalosporins, fluoroquinolones, and beta-lactam/beta-lactamase inhibitor combinations. Since clindamycin was rarely used in our two hospital study (‹4% of discharges), this antibiotic class, which has been previously identified as high risk for CDI, was not included in our primary exposure variable. In additional analyses, we also compared patients with exposure to low-risk antibiotics (i.e., any antibiotic not included in the high-risk group) to patients without exposure to antibiotics.
We conducted univariate comparisons of important variables using t-tests or Wilcoxon Rank tests for continuous variables and Chi Square or Fisher’s Exact tests for categorical variables as applicable.
In the multivariate analysis, we employed a logistic model. From the model, we estimated the risk ratio controlling for age, sex, Gagne co-morbidity score (1), hospital, and CDI pressure (2). To determine CDI pressure, patients identified as having CDI in the EIP were considered infectious for 14 days following the initial EIP diagnosis date. Then for each calendar date during the timeframe of the study, the number of infectious CDI patients by hospital ward was determined. Each cohort participant’s CDI pressure was estimated by determining the number of infectious patients for each day that the hospitalized patient spent in the same hospital ward location as another patient(s) in the cohort with active CDI (2). The number of infectious patients was then summed for the hospitalized patient’s entire stay. Only days prior to patient’s CDI infection were included in this measure.
RESULTS:
At the two hospitals, 35,567 index visits were observed with a total of 412 CDI cases (11.6 per 1,000 discharges). The unadjusted risk among those exposed to high-risk antibiotics was higher compared to all others (RR=3.7, 95% CI: 3.0-4.5). In the multivariate logistic model, we estimated the RR comparing those exposed to high-risk antibiotics to those with low-risk or no antibiotic exposure. In our model, the risk of CDI among those exposed to high-risk antibiotics was 3 times higher compared to persons with low-risk or no antibiotic exposure (RR=2.9, 95% CI: 2.3-3.5). Further, we generated additional estimates comparing exposure to high-risk or low-risk antibiotics to no antibiotics for hospital onset (0 days follow-up) as well as 42 and 180 days of follow-up for post-discharge CDI, (see Table 1).
Risk of Clostridium difficile Infection (Hospital Onset and Post-Discharge) following High-Risk or Low-Risk Antibiotics compared to No Antibiotic Exposure | ||||||
---|---|---|---|---|---|---|
# Days in Post-Discharge Window | ||||||
0 (Hospital Onset) | 42 | 180 | ||||
RR* Low-Risk Antibiotics† vs No Antibiotics | 1.5 | 0.8-2.7 | 1.3 | 0.8-1.9 | 1.0 | 0.7-1.3 |
RR* High-Risk Antibiotics† vs No Antibiotics | 3.6 | 2.3-5.6 | 3.1 | 2.3-4.2 | 2.8 | 2.2-3.6 |
*Data from two large academic centers participating in the Emerging Infections Program. Adjusted for gender, age, Gagne co-morbidity score, CDI pressure, hospital. RR: Relative risk; results expressed as a point estimate followed by the 95% confidence interval. | ||||||
†High risk antibiotics were defined as: 3rd/4th generation cephalosporins; fluoroquinolones; beta-lactam/beta-lactamase inhibitor combinations. All other antibiotics were considered to be low-risk. |
DISCUSSION:
Limitations
There were several limitations in this analysis. First, administrative data was used to establish the exposures, and there is a potential for misclassification. However, we do not expect the misclassification to be differential, and therefore any effect would likely bias our estimates towards the null. Second, only two hospitals were available. The relative risk identified in the study may not be generalizable, and future studies in additional populations could address this limitation. However, past studies have also identified a similar level of risk (3, 4). Third, we do not have additional information on outpatient exposures, especially outpatient antibiotic prescriptions. Determining the effect of outpatient antibiotic exposures on our estimates without additional information on outpatient prescribing patterns for hospitalized patients is difficult. In addition, as our study was limited to adults, our estimates would only apply to adult hospitalized populations. Because CDI is less common in pediatric populations, we focused this study on only adult patients. Additional models that allow for time-dependent covariates for our primary exposure variable, antibiotics, may also be valuable in quantifying the excess risk related to certain antibiotics.
Strengths
Our study had a number of strengths. First, confirmed CDI cases from EIP surveillance linked to hospital administrative data were used. Therefore, our outcome did not rely on ICD-9-CM codes which do not always reliably identify incident cases of CDI. Further, use of the EIP surveillance data allowed the capture of not only those cases identified during a hospital stay, but also those having their onset post-discharge. There were a large number of index hospitalizations, greater than 35,000, included in the study. In addition, our hospital data included pharmacy data as well; which is not always readily available from large hospital data sets.
Conclusions
In conclusion, our study identified a ~3 times higher risk of hospital-onset and post-discharge CDI among those exposed to high-risk antibiotics during a hospitalization visit.
Estimating Antibiotic Usage in a Sample of 323 US Hospitals from the MarketScan® Hospital Drug Database
METHODS:
We retrospectively estimated the proportion of hospital discharges in which a patient received at least one dose of an antibiotic during their stay. Adult and pediatric drug use data was obtained from the Truven Health MarketScan® Hospital Drug Database (HDD). The HDD contains individual billing records for all patients from approximately 600 hospitals. For this analysis, we included hospital admissions for all patients discharged from January 1, 2010 through December 31, 2010. For each discharge, we identified antibiotic doses administered from the drug utilization data, which is based on charge data and includes details from internal drug and supply systems. From the details in the drug utilization data, we also assigned an antibiotic class. Specific antibiotics and their respective classes were identified from multiple sources including the HDD, available information from the US Food and Drug Agency (http://www.fda.gov/Drugs/InformationOnDrugs/ucm142438.htm), and the World Health Organization (http://www.whocc.no/). We eliminated drugs with non-oral, non-parental routes of administration (including topical, ophthalmic, dental, intrathecal, otic, vaginal, rectal, nasal, and urogenital). We further eliminated any hospital whose data did not identify any antibiotics administered at the hospital. Both facilities and patient level data in the HDD are non-identifiable.
Once we identified discharges with an antibiotic administered and the one or more classes of antibiotics administered during the stay, we determined the proportion of all discharges in which an antibiotic was administered during at least one hospital day, both overall and stratified by class, using the available hospital discharge data. We also estimated the proportion of discharges with at least one day of antibiotic therapy considered to pose a high-risk for subsequent development of CDI. High-risk antibiotics were defined as 3rd/4th generation cephalosporins, fluoroquinolones, and beta-lactam/beta-lactamase inhibitor combinations. Since clindamycin was rarely used among participating hospitals (<4% of discharges), this antibiotic class, which has been previously identified as high risk for CDI, was not included as a high-risk antibiotic. Patient demographic characteristics such as age were obtained from the HDD. We generated estimates for all pediatric and adult patients as well as adults only (>17 years). Finally, we estimated the number of antibiotic days of therapy among all inpatient hospital days for the compartmental models described below. Results are described in the Vital Signs.
Stochastic Model to Estimate the Potential Reductions in Clostridium difficile infection
METHODS:
Model overview
To investigate the relative risk between high- and low-risk inpatient antibiotic exposures for the outcome of CDI, we performed a computer simulation. We used the same definition of high-risk antibiotics as the observational study described above: 3rd/4th generation cephalosporins, fluoroquinolones, and beta-lactam/beta-lactamase inhibitor combinations. Since clindamycin was rarely used in our two hospital study (<4% of discharges), this antibiotic class, which has been previously identified as high risk for CDI, was not included in our primary exposure variable. A stochastic, compartmental model was used to incorporate antibiotic exposure, transmission, progression to disease, and treatment for patients in a single, virtual hospital. A total of twenty compartments were used to represent composite patient states, including both current and recent antibiotic exposure, as well as C. difficile carriage and CDI status. Antibiotic exposure was modeled in two dimensions: whether the patient had no exposure, current exposure, or recent exposure; and whether the exposure was to high- or low-risk antibiotics. Five compartments were used to describe these possible states. There were four possible states reflecting carriage and disease status. Patients were either susceptible to colonization with C. difficile, asymptomatically colonized, had active CDI, or had a history of resolved CDI.
Model details
Upon admission to the hospital, patients were randomly assigned to being on or off antibiotics at the time of admission. Additionally, patients admitted to the hospital were randomly assigned to one of four carriage and disease states. All probabilities that governed the admission compartments were based on values used in previous work (5).
The rate that patients not on antibiotics were prescribed antibiotics was estimated through model calibration. Once patients were put on antibiotics, antibiotics were discontinued at a rate consistent with the observed values of mean antibiotic exposure. When antibiotics were discontinued, patients’ risk for CDI remained elevated through the post-antibiotic period which lasted until discharge.
Patients who were susceptible to colonization became colonized at a rate assuming mass action dynamics and that the incidence of transmission was proportional to the density of infectious individuals. This rate was modified by susceptibility and transmissibility parameters, which were in turn influenced by patients’ antibiotic status (not on antibiotics, on high-risk, on low-risk, post-high-risk, post-low-risk). Once colonized, patients progressed to symptomatic CDI based on disease progression rates, which also depended on antibiotic status.
Patients could be discharged from any state. Discharge rates were calibrated to correspond with an overall mean length of stay of about 5 days, and patients with CDI were discharged much less frequently so that almost all CDI patients remained in the hospital until their symptoms were resolved.
Calibration
Most parameter values were based on those used in previous work (Rubin 2013). However, some parameters in this model needed to be calibrated to reflect observed calibration targets from the EIP and MarketScan® Hospital Drug Database (HDD) studies described above. There were four principal calibration targets:
- C1) Ratio of antibiotic days to patient days
- C2) Ratio of high-risk antibiotic days to antibiotic days
- C3) Risk ratio of infection for low-risk antibiotic exposure to no antibiotic exposure
- C4) Risk ratio of infection for high-risk antibiotic exposure to no antibiotic exposure
The most critical parameter values that were explored that had the most dominant impact on the four calibration targets were:
- P1) Transmissibility (not on antibiotics, on high- or low-risk antibiotics, post-high- or low-risk antibiotics)
- P2) Susceptibility (not on antibiotics, on high- or low-risk antibiotics, post-high- or low-risk antibiotics)
- P3) Rates of progression to disease (not on antibiotics, on high- or low-risk antibiotics, post-high- or low-risk antibiotics)
- P4) Transition to high- or low-risk antibiotics
A broad range of possible values were selected for each parameter resulting in many parameter combinations being used for calibration. From this parameter exploration, reasonable ranges for each of the parameters were identified as being consistent with the four calibration targets.
Experiment
The primary outcome for the simulation experiment was hospital-onset CDI rates (infections per 1000 patient days). The main experiment consisted of running the simulation under “normal” high-risk antibiotic rates (i.e., high-risk antibiotic rates consistent with the studies described above) and then running the model under the same parameterization with the exception of an approximate 30% reduction in the number of patients receiving high-risk antibiotics. This provided a framework for comparing the two scenarios. To account for the stochastic nature of the computer simulation, we performed 500 independent simulations with each framework in the experiment (“normal” high-risk antibiotic rates and a 30% reduction in high-risk antibiotics). To account for uncertainty in the parameter values, whose ranges were selected in the calibration step, each simulation used parameter values randomly drawn from a uniform distribution yielding values within the consistent range previously identified. Each simulation assumed a 300 bed hospital and generated data over a 10 year period. Although the simulations reached equilibrium within two weeks, the first 30 days of simulated data were excluded from the analysis to account for a burn-in period. Results are described in full in the Vital Signs and include the total direct and indirect effects (6).
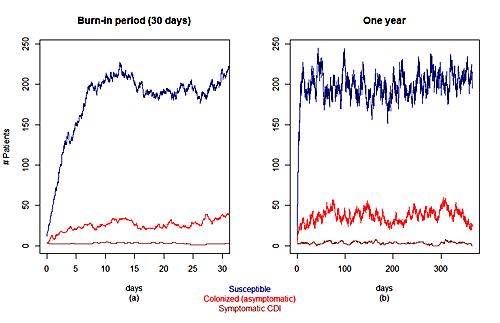
Plots showing the variation over time of the numbers of susceptible, colonized and asymptomatic, and symptomatic patient changes during: (a) the first 30 days in the simulation (b) a one year period
References
- Gagne JJ, Glynn RJ, Avorn J, Levin R, Schneeweiss S. A combined comorbidity score predicted mortality in elderly patients better than existing scores. Journal of clinical epidemiology. 2011 Jul;64(7):749-59. PubMed PMID: 21208778. Pubmed Central PMCID: 3100405.
- Dubberke ER, Reske KA, Olsen MA, McMullen KM, Mayfield JL, McDonald LC, et al. Evaluation of Clostridium difficile-associated disease pressure as a risk factor for C difficile-associated disease. Archives of internal medicine. 2007 May 28;167(10):1092-7. PubMed PMID: 17533213.
- Hensgens MP, Goorhuis A, Dekkers OM, Kuijper EJ. Time interval of increased risk for Clostridium difficile infection after exposure to antibiotics. The Journal of antimicrobial chemotherapy. 2012 Mar;67(3):742-8. PubMed PMID: 22146873.
- Slimings C, Riley TV. Antibiotics and hospital-acquired Clostridium difficile infection: update of systematic review and meta-analysis. The Journal of antimicrobial chemotherapy. 2013 Dec 8. PubMed PMID: 24324224.
- Rubin MA, Jones M, Leecaster M, Khader K, Ray W, Huttner A, et al. A simulation-based assessment of strategies to control Clostridium difficile transmission and infection. PloS one. 2013;8(11):e80671. PubMed PMID: 24278304. Pubmed Central PMCID: 3836736.
- Lipsitch M, Samore MH. Antimicrobial use and antimicrobial resistance: a population perspective. Emerging infectious diseases. 2002 Apr;8(4):347-54. PubMed PMID: 11971765. Pubmed Central PMCID: 2730242.
- Page last reviewed: April 7, 2015
- Page last updated: April 7, 2015
- Content source: