Early Onset Obesity and Risk of Metabolic Syndrome Among Chilean Adolescents
ORIGINAL RESEARCH — Volume 14 — October 12, 2017
Lorena Sonia Pacheco, MPH, RDN, CPH1; Estela Blanco, MPH, MA2,3; Raquel Burrows, MD4; Marcela Reyes, MD, PhD4; Betsy Lozoff, MD, MS5,6; Sheila Gahagan, MD, MPH2,5 (View author affiliations)
Suggested citation for this article: Pacheco LS, Blanco E, Burrows R, Reyes M, Lozoff B, Gahagan S. Early Onset Obesity and Risk of Metabolic Syndrome Among Chilean Adolescents. Prev Chronic Dis 2017;14:170132. DOI: http://dx.doi.org/10.5888/pcd14.170132.
PEER REVIEWED
Abstract
Introduction
Obesity and metabolic syndrome (MetS) indicators have increased globally among the pediatric population. MetS indicators in the young elevate their risk of cardiovascular disease and metabolic disorders later in life. This study examined early onset obesity as a risk factor for MetS risk in adolescence.
Methods
A cohort of Chilean participants (N = 673) followed from infancy was assessed at age 5 years and in adolescence (mean age, 16.8 y). Adiposity was measured at both time points; blood pressure and fasting blood samples were assessed in adolescence only. Early onset obesity was defined as a World Health Organization z score of 2 standard deviations (SDs) or more for body mass index (BMI) at age 5 years. We used linear regression to examine the association between early onset obesity and adolescent MetS risk z score, adjusting for covariates.
Results
Eighteen percent of participants had early onset obesity, and 50% of these remained obese in adolescence. Mean MetS risk z score in adolescence was significantly higher among those with early onset obesity than among those without (1.0; SD, 0.8 vs 0.2; SD, 0.8 [P < .001]). In the multivariable model, early onset obesity independently contributed to a higher MetS risk score in adolescence (β = 0.27, P < .001), controlling for obesity status at adolescence and sex, and explained 39% of the variance in MetS risk.
Conclusion
Early onset obesity as young as age 5 years relates to higher MetS risk.
Introduction
Obesity among children is a global public health problem (1,2), and signs of metabolic syndrome (MetS) have increased among both children and adolescents over the past 25 years (3,4). MetS is defined as having at least 3 of 5 risk factors: a large waist circumference, high blood pressure, fasting hyperglycemia, hypertriglyceridemia, and low high-density lipoprotein (HDL) cholesterol levels (5). Although few children meet all 5 MetS criteria, up to 30% of obese children have at least one element of MetS (6). A recent systematic review showed a median prevalence of MetS of 3% (range 0%–19.2%) among all children and 29% (range 10%–66%) among obese children (3). In a sample of US adolescents aged 12 to 17 years, the overall MetS prevalence was 7%, with a range from 19% to 35% among obese adolescents (7). Additionally, Hispanic adolescents had a higher MetS prevalence (11%) than non-Hispanic white adolescents (9%) (8).
MetS increases a person’s risk for developing chronic disease (9,10). Pediatric MetS is independently associated with type 2 diabetes and adult MetS and with subclinical atherosclerosis leading to cardiovascular disease (CVD) (11,12). Additionally, research shows that obesity tracks from childhood into adulthood (13) and contributes to adverse consequences, including premature mortality and cardiometabolic disorders (14,15). However, the impact of the age of obesity onset in early childhood on adolescent MetS risk has not been documented, because research has largely focused on infant weight gain and catch-up growth as predictors of health outcomes later in life (16–18). This study examined early onset obesity as a risk factor for MetS risk in adolescence. We hypothesized that obesity onset early in life is associated with a higher MetS risk score in adolescence.
Methods
Study design and population
Participants were 677 Chilean infants who were part of an observational longitudinal study of biopsychosocial determinants of obesity and CVD risk. From 1991 through 1996, 1,933 infants were enrolled in either a preventive trial of iron supplementation to prevent iron-deficiency anemia or a neuromaturation study, a study that assessed neurodevelopment by using neurophysiological and electrophysiological techniques. The studies were conducted in Santiago, Chile, where infancy iron deficiency was widespread at the time and no national program existed for iron supplementation. Infants were from low-to-middle income, working-class communities. Inclusion criteria for the infancy studies were an uncomplicated, singleton, term, vaginal birth with birthweights of 3 kg or more, no major congenital abnormalities, and no prior iron therapy. Because of a successful national breastfeeding campaign, all but 8 infants in the cohort were initially breastfed.
Infants were recruited at age 4 to 6 months. Infants without iron-deficiency anemia were randomly assigned to high-iron supplementation, low-iron supplementation, or usual nutrition (no added iron). Further details about enrollment and trial specifications are described elsewhere (19). A total of 1,657 infants completed the preventive trial (high iron, n = 718; low iron, n = 405; usual nutrition, n = 534). Infants found to have iron-deficiency anemia, and the next nonanemic infant (the control), were treated with medicinal iron and participated the neuromaturation study (20). A total of 135 infants completed the neuromaturation study. At age 5 years, because of a cut in funding, only 2 of the 3 randomly selected preventive trial groups and neuromaturation trial participants could be evaluated. Thus, only 888 of 1,501 infants who were in the high-iron and no-added-iron groups or completed the neuromaturation study were assessed. At age 16 years, participants from the 5-year follow up were invited to participate in a study of obesity and CVD risk. A total of 677 of 888 (76%) participants agreed and were assessed from 2009 through 2012. Our analytic sample for the obesity and MetS study consisted of 673 participants from the obesity and CVD risk study who had complete data at 5 years and 16 years (Figure).
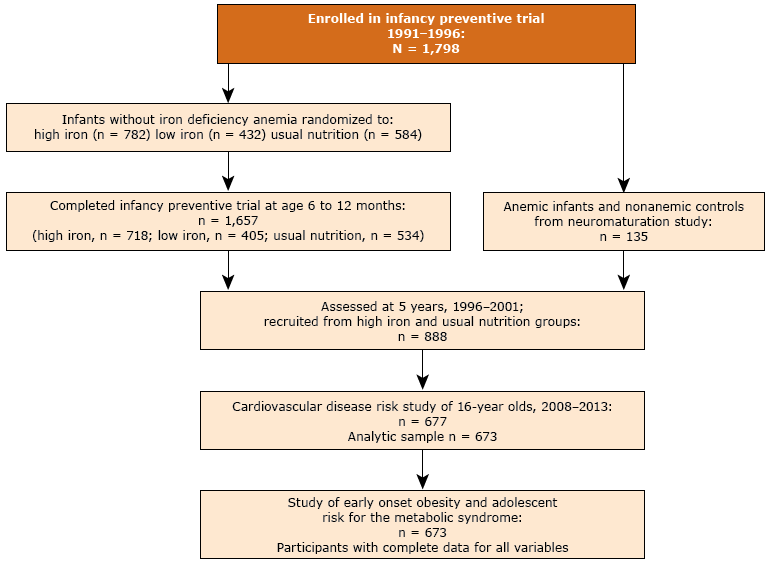
Figure.
Flow of participants in study on relationship between early onset obesity and metabolic syndrome risk in adolescence, Santiago, Chile, 2009–2012. Participants were drawn from a larger study of infancy iron-deficiency anemia. [A text version of this figure is also available,]
The sample was representative of the original cohort, with no differences in infant and family characteristics, including birthweight (3.5 kg in the original cohort vs 3.6 kg in the final analytic sample), breastfeeding for at least 6 months (63% in the original cohort vs 61% in the final analytic sample), socioeconomic status (SES) (27.3 on the Graffar index [21] for the original cohort vs 27.0 for the final analytic sample), and household environment (30.1 on the Home Observation for Measurement of the Environment [HOME] [22] scale for the original cohort vs 30.0 for the final analytic sample). The study was approved by the institutional review boards of the University of California, San Diego, the University of Michigan, and the University of Chile Institute of Nutrition and Food Technology (INTA).
Data collection and analysis
Participants were considered to have early onset obesity if obesity was present at age 5 years (defined as ≥2 standard deviations [SDs] for body mass index [BMI] z score) by using WHO growth standard indicators. BMI is a measure of weight relative to height (kg/m2), with age-specific and sex-specific norms (23).
Adolescents were assessed at age 16 to 17 years. Height (cm), weight (kg), waist and hip circumference (cm), and blood pressure (mmHg) were measured by a physician-investigator at INTA. Standardized procedures were used to measure weight to the closest 0.1 kg by using a SECA scale (SECA), and height to the closest 0.1 cm by using a Holtain stadiometer (Holtain Ltd) (24). Each measurement was taken twice, and a third measurement was taken if the difference between the first 2 exceeded 0.3 kg for weight, 0.5 cm for height, or 1.0 cm for waist. The WHO BMI z score indicator was used to dichotomize (yes/no) obesity status at adolescence, with obesity defined as an SD of 2 or more in the BMI z score. Fasting serum triglyceride, cholesterol, and glucose levels were assessed. Serum glucose concentration (mg/dL) was determined by using an enzymatic–colorimetric test (Química Clínica Aplicada S.A.). Triglyceride (mg/dL), and cholesterol (mg/dL) levels were determined with the Vitros dry analytical methodology (Ortho Clinical Diagnostics Johnson & Johnson Inc).
A continuous MetS risk z score was calculated by applying the equations developed by Gurka et al (25). The equations provide a sex-specific and race-specific z score measure for MetS risk based on standardized and log-transformed values for each component of the MetS.
Characteristics that may be associated with both the variable of interest and the outcome were considered covariates. For infancy, the following were considered: birthweight, SES, breastfeeding, emotional and material support provided in the home environment, iron status during infancy, and iron supplementation as part of the preventive trial. For adolescents, the following were potential covariates: age at menarche, age at the adolescent assessment, physical activity, and obesity status. Birthweight, measured in kilograms, was analyzed as a continuous measure. Iron status during infancy, coded as iron sufficient, iron deficient, or iron-deficient anemic, was dichotomized to iron sufficient (0) and iron deficient or iron-deficient anemic (1) for modeling purposes. Iron supplementation in infancy was dichotomized to iron supplementation (high or low) (1) and no iron supplementation (0). The SES variable, the Graffar index, is a pseudocontinuous variable based on a 13-item questionnaire that produces a composite score that comprises questions on mothers’ and fathers’ years of education, occupation, and income (21) the higher the Graffar index, the lower the SES. Questions were coded as absent (1) to plentiful (6), for a possible score range of 13 to 78. The quality of the home environment that supported the children’s development was assessed with HOME, a 45-item, observer-rated checklist (22). A higher HOME score reflects a more supportive home environment for children’s development. Scores range from 0 to 45. Physical activity at adolescence was measured by using a 5-item questionnaire, validated for use in young populations (26). The questionnaire addresses planned and unplanned physical and sedentary activities as a continuous score between 0 and 10. Age at menarche and age at adolescent measurement were analyzed as continuous variables.
Statistical analyses
SAS version 9.2 (SAS Institute) was used for all statistical analyses, with the exception of computed MetS risk score, for which SPSS version 22.0 (IBM Corp) was used.
For describing sample characteristics, continuous variables were expressed as mean and SD, and categorical variables were expressed as frequencies. All variables were assessed for normality. Unadjusted comparisons between early onset obesity groups were calculated by using t tests for continuous outcomes and χ2 tests for categorical outcomes. Regression diagnostics, using tests and graphical methods, examined linear regression assumptions including linearity (residual vs predictor plot) normality (Shapiro–Wilk test), homogeneity of variance (Breusch–Pagan test) and independence (Durbin–Watson statistic). All of these assumptions were met, indicating linearity, uncorrelated and normally distributed estimated residuals with constant variance. Influence and collinearity were also examined, with no extreme deviations observed for studentized and jackknife residuals, and small leverage and Cook’s distance values. Multivariable linear regression analysis was used to assess the relationship between early onset obesity and MetS risk score, adjusting for possible confounders. The full model was tested by using backward elimination. Variables that were not statistically associated with the dependent variable were manually removed. Because the study sample participated in a preventive trial, iron status during infancy and iron supplementation were initially included as covariates. Neither variable was significantly associated with the outcome, and thus both were removed from the final model. Age at menarche, which was tested in the multivariable model, was not significantly associated with outcome and therefore was dropped from the final model. Significance was set at a P value of less than .05. Multicollinearity between variables was assessed with tolerance level with a cut point of less than 0.10. There was no evidence of multicollinearity in the model.
Marginal structural models (MSMs) refine the adjustment made by traditional analytic approaches and predict an estimate that accounts for the bias that exists when time-dependent covariates might act as both confounders and intermediates in a linear association. We used an MSM as a sensitivity analysis to account for potential bias resulting from the inclusion of adolescent weight status in the final model; bias may arise because adolescent weight status both mediates and confounds the relationship between early-life obesity and adolescent MetS risk score. To carry out these analyses, we estimated stabilized inverse probability weights (27) and reweighted our sample to create a pseudopopulation in which the exposure, early onset obesity, is statistically independent of potential time-dependent confounders. Results from the pseudopopulation models supported initial findings, indicating limited bias resulting from the inclusion of adolescent weight status as a covariate in the multivariable linear regression model.
Results
Mean age of participants at adolescence was 16.8 years, and 52.9% were male (Table 1). The mean birthweight in the study population was 3.6 kg (SD, 0.4 kg). Early onset obesity was found in 18.1% of the participants, of which 41.0% were girls. We found no significant differences in birthweight, sex, SES, HOME scores, and physical activity in adolescence between participants with early onset obesity and participants without early onset obesity. Obesity status at adolescence was related to early onset obesity. Of those with early onset obesity, 50% were obese at adolescence, in contrast to 6% of the comparison group (P < .001).
The MetS risk score and all variables related to CVD risk were significantly higher in the early onset obesity group, compared with the group without early onset obesity, with the exception of HDL cholesterol, which was inversely related to CVD risk, and fasting blood glucose, which did not differ between groups (Table 2). Participants in the early onset obesity group had significantly higher mean total cholesterol levels (156.4 mg/dL; SD, 27.9 vs 151.1 mg/dL; SD 27.5, P = .04) and low-density lipoprotein cholesterol levels (98.9 mg/dL, SD 24.8 vs 93.3 mg/dL, SD 24.2, P = .03) compared with the group without early onset obesity. Additional analyses for MetS components by sex showed that adolescent boys had significantly lower mean total cholesterol and HDL cholesterol levels and significantly higher fasting blood glucose levels and blood pressure than adolescent girls.
The final model, controlling for sex and obesity status in adolescence, indicated that early onset obesity was associated with a higher MetS risk score in adolescence (β = 0.27; 95% confidence interval [CI] 0.13– 0.41, P < .001) (Table 3) and explained 39% of the variance in the MetS risk score. The adjusted mean and standard error (SE) MetS risk score was 1.0 (SE, 0.06) and 0.7 (SE, 0.04) for participants with and without early onset obesity, respectively. Additionally, female sex was associated with a lower MetS risk score in the model (β = −0.26; 95% CI, −0.35 to −0.17; P < .001), adjusting for other covariates.
Findings from the MSM, a sensitivity analysis, did not differ from findings of the multivariable regression analysis. This corroborated the effect size of early onset obesity and its relationship with MetS risk score in adolescence.
Discussion
This study showed that early onset obesity was associated with greater MetS risk in adolescence. Independent of adolescent obesity status and sex, a child who had obesity at age 5 years had a higher MetS risk score (β = 0.27) at age 16. These results support our initial hypothesis.
Our findings are consistent with those in a mid-childhood cohort (28). Using a similar analytic approach and focusing on metabolic profiles that included dyslipidemia, hypertension, and insulin resistance, Garnett et al. (28) concluded that children who were overweight or obese at age 8 years were almost 7 times as likely to have CVD risk-clustering at age 15 years as those who were not overweight or obese (odds ratio, 6.9; 95% CI, 2.5– 19.0; P < .001) (28).
Boys in our cohort had higher mean MetS risk scores than girls, independent of early onset obesity and obesity status at adolescence. These results are similar to our prior findings (29) and those of US national data, in which adolescent boys were more likely to have MetS risk factors than adolescent girls (9). A recent systematic review of the prevalence of MetS in children and adolescents from 12 countries in North America and South America also found a higher prevalence of MetS among boys (29). Adolescent boys also manifested higher fasting blood glucose, higher blood pressure, and lower HDL cholesterol levels than adolescent girls. These CVD risks were primarily observed in Mexico, Canada, Colombia, and the United States (30).
This research emphasizes the value of studying longitudinal cohorts and the relevance this study’s cohort to obesity in early childhood and adolescent health outcomes among Chileans. In addition to the longitudinal study design, this study has several strengths, such as the uniqueness of a Chilean cohort of infants followed successfully to adolescence, inclusion of a relatively large group of healthy infants, and good participant retention. Another strength is that the evaluation was conducted at a nutrition research center by highly trained study personnel. Furthermore, the use of a sex-specific and race-specific continuous MetS risk score is a study strength. Although continuous scores were previously developed, the methodology followed by Gurka et al and applied in this study, acknowledges correlations between the MetS components, accounting for MetS component correlation differences by sex and race/ethnicity (25).
A limitation of this study is that it may not be generalizable to other populations. The participant sample was restricted to infants who weighed 3 kg or more at birth. Thus, we cannot infer whether these relationships translate to preterm or low-birthweight infants. Also, probably because data on birthweight were restricted, we probably did not observe a relationship between birthweight and obesity. Generalization to higher-income or poverty groups is also restricted. Another limitation is lack of anthropometric data between measurement waves, thus placing participants in a BMI category at time of measurement, which might have been different a year before or after measurement. Additionally, data were unavailable on maternal or paternal obesity status, diet intake, and direct physical activity measures. Although we attempted to minimize unmeasured confounding in our study by including measures on recognized potential confounders, such unmeasured risk factors could confound the relationship between early onset obesity and MetS risk.
Notwithstanding these limitations, our findings add to the literature on early life determinants, in particular determinants related to the long-term effects of early onset obesity on MetS or other CVD-related risk factors. Future research should be conducted in populations with various races and ethnicities to substantiate these findings and address a key public health problem.
Our results underscore the public health implications of early childhood obesity for health outcomes later in life. The findings provide evidence for a clinically meaningful and significant association between early onset obesity and MetS risk score in this Chilean cohort. The results of this study emphasize the importance and need for early detection of childhood obesity and effective public health interventions.
Acknowledgments
The project was supported by grants from the National Heart, Lung, and Blood Institute (R01HL088530, principal investigator, Sheila Gahagan) and the National Institute of Child Health and Human Development (R01HD14122, principal investigator, Betsy Lozoff, and R01HD33487, principal investigators, Betsy Lozoff and Sheila Gahagan). The authors thank the study participants and their families for their continuous involvement.
Author Information
Corresponding Author: Sheila Gahagan, MD, MPH, Department of Pediatrics, University of California, San Diego, 9500 Gilman Dr, MC 0927, La Jolla, CA 92093-0927. Telephone: 619-681-0662. Email: sgahagan@ucsd.edu.
Author Affiliations: 1University of California San Diego–San Diego State University Joint Doctoral Program in Public Health, La Jolla, California. 2Division of Child Development and Community Health, Department of Pediatrics, University of California, San Diego, California. 3University of Chile Doctoral Program in Public Health, Santiago, Chile. 4Public Health Nutrition Unit, Institute of Nutrition and Food Technology (INTA), University of Chile, Santiago, Chile. 5Center for Human Growth and Development, University of Michigan, Ann Arbor, Michigan. 6Department of Pediatrics and Communicable Diseases, University of Michigan, Ann Arbor, Michigan.
References
- Karnik S, Kanekar A. Childhood obesity: a global public health crisis. Int J Prev Med 2012;3(1):1–7. PubMed
- Rivera JA, de Cossío TG, Pedraza LS, Aburto TC, Sánchez TG, Martorell R. Childhood and adolescent overweight and obesity in Latin America: a systematic review. Lancet Diabetes Endocrinol 2014;2(4):321–32. CrossRef PubMed
- Friend A, Craig L, Turner S. The prevalence of metabolic syndrome in children: a systematic review of the literature. Metab Syndr Relat Disord 2013;11(2):71–80. CrossRef PubMed
- Lee L, Sanders RA. Metabolic syndrome. Pediatr Rev 2012;33(10):459–66, quiz 467–8. CrossRef PubMed
- Alberti KG, Eckel RH, Grundy SM, Zimmet PZ, Cleeman JI, Donato KA, et al. ; International Diabetes Federation Task Force on Epidemiology and Prevention; Hational Heart, Lung, and Blood Institute; American Heart Association; World Heart Federation; International Atherosclerosis Society; International Association for the Study of Obesity. Harmonizing the metabolic syndrome: a joint interim statement of the International Diabetes Federation Task Force on Epidemiology and Prevention; National Heart, Lung, and Blood Institute; American Heart Association; World Heart Federation; International Atherosclerosis Society; and International Association for the Study of Obesity. Circulation 2009;120(16):1640–5. CrossRef PubMed
- Owens S, Galloway R. Childhood obesity and the metabolic syndrome. Curr Atheroscler Rep 2014;16(9):436–44. CrossRef PubMed
- Kiess W, Blüher S, Kapellen T, Körner A. Metabolic syndrome in children and adolescents: prevalence, public health issue, and time for initiative. J Pediatr Gastroenterol Nutr 2009;49(3):268–71. CrossRef PubMed
- Laurson KR, Welk GJ, Eisenmann JC. Diagnostic performance of BMI percentiles to identify adolescents with metabolic syndrome. Pediatrics 2014;133(2):e330–8. CrossRef PubMed
- Johnson WD, Kroon JJ, Greenway FL, Bouchard C, Ryan D, Katzmarzyk PT. Prevalence of risk factors for metabolic syndrome in adolescents: National Health and Nutrition Examination Survey (NHANES), 2001–2006. Arch Pediatr Adolesc Med 2009;163(4):371–7. CrossRef PubMed
- Grundy SM. Metabolic syndrome pandemic. Arterioscler Thromb Vasc Biol 2008;28(4):629–36. CrossRef PubMed
- Morrison JA, Friedman LA, Gray-McGuire C. Metabolic syndrome in childhood predicts adult cardiovascular disease 25 years later: the Princeton Lipid Research Clinics Follow-up Study. Pediatrics 2007;120(2):340–5. CrossRef PubMed
- DeBoer MD, Gurka MJ, Woo JG, Morrison JA. Severity of the metabolic syndrome as a predictor of type 2 diabetes between childhood and adulthood: the Princeton Lipid Research Cohort Study. Diabetologia 2015;58(12):2745–52. CrossRef PubMed
- de Onis M, Blössner M, Borghi E. Global prevalence and trends of overweight and obesity among preschool children. Am J Clin Nutr 2010;92(5):1257–64. CrossRef PubMed
- Singh AS, Mulder C, Twisk JW, van Mechelen W, Chinapaw MJ. Tracking of childhood overweight into adulthood: a systematic review of the literature. Obes Rev 2008;9(5):474–88. CrossRef PubMed
- Reilly JJ, Kelly J. Long-term impact of overweight and obesity in childhood and adolescence on morbidity and premature mortality in adulthood: systematic review. Int J Obes 2011;35(7):891–8. CrossRef PubMed
- Fagerberg B, Bondjers L, Nilsson P. Low birth weight in combination with catch-up growth predicts the occurrence of the metabolic syndrome in men at late middle age: the Atherosclerosis and Insulin Resistance study. J Intern Med 2004;256(3):254–9. CrossRef PubMed
- Ekelund U, Ong KK, Linné Y, Neovius M, Brage S, Dunger DB, et al. Association of weight gain in infancy and early childhood with metabolic risk in young adults. J Clin Endocrinol Metab 2007;92(1):98–103. CrossRef PubMed
- Barker DJ. Sir Richard Doll Lecture. Developmental origins of chronic disease. Public Health 2012;126(3):185–9. CrossRef PubMed
- Lozoff B, De Andraca I, Castillo M, Smith JB, Walter T, Pino P. Behavioral and developmental effects of preventing iron-deficiency anemia in healthy full-term infants. Pediatrics 2003;112(4):846–54. PubMed
- Roncagliolo M, Garrido M, Walter T, Peirano P, Lozoff B. Evidence of altered central nervous system development in infants with iron deficiency anemia at 6 mo: delayed maturation of auditory brainstem responses. Am J Clin Nutr 1998;68(3):683–90. PubMed
- Mendez-Castellano H, Mendez MC. Estratificación social y biología Estratificación social y humana: método Graffar modificado/social stratification and human biology: Graffar’s modified method. Arch Venez Pueric Pediatr 1986;49:93–104.
- Bradley RH, Corwyn RF, McAdoo HP, Coll CG. The home environments of children in the United States part I: variations by age, ethnicity, and poverty status. Child Dev 2001;72(6):1844–67. CrossRef PubMed
- World Health Organization Multicentre Growth Reference Study Group. WHO child growth standards: growth velocity based on weight, length and head circumference. Methods and development. Geneva (CH): World Health Organization; 2009.
- Lohman TG, Roche AF, Martorell R. Anthropometric standardization reference manual. Champaign (IL): Human Kinetics Books; 1988. vi, p.177.
- Gurka MJ, Ice CL, Sun SS, Deboer MD. A confirmatory factor analysis of the metabolic syndrome in adolescents: an examination of sex and racial/ethnic differences. Cardiovasc Diabetol 2012;11(1):128–38. CrossRef PubMed
- Godard M C, Rodríguez N MP, Díaz N, Lera M L, Salazar R G, Burrows A R. Valor de un test clínico para evaluar actividad física en niños. [Value of a clinical test for assessing physical activity in children]. Rev Med Chil 2008;136(9):1155–62. PubMed
- Robins JM, Hernán MA, Brumback B. Marginal structural models and causal inference in epidemiology. Epidemiology 2000;11(5):550–60. CrossRef PubMed
- Garnett SP, Baur LA, Srinivasan S, Lee JW, Cowell CT. Body mass index and waist circumference in midchildhood and adverse cardiovascular disease risk clustering in adolescence. Am J Clin Nutr 2007;86(3):549–55. PubMed
- Burrows R, Correa-Burrows P, Reyes M, Blanco E, Albala C, Gahagan S. High cardiometabolic risk in healthy Chilean adolescents: associations with anthropometric, biological and lifestyle factors. Public Health Nutr 2016;19(3):486–93. CrossRef PubMed
- Pierlot R, Cuevas-Romero E, Rodriguez-Antolin J, Mendez-Hernandez P, Martinez-Gomez M. Prevalencia de Sindrome Metabolico en niños y adolescentes de America. TIP: Revista Especializada en Ciencias Químico-Biológicas 2017;20(1):40–9. CrossRef
Tables
Table 1. Characteristics of Participants (N = 673), Study of Relationship Between Early Onset Obesity and Risk of Metabolic Syndrome Among Adolescentsa, Santiago, Chile, 1991–1996 and 2009–2012
Characteristic | Total Sample (n = 673) | Early Onset Obesity (n = 122) | No Early Onset Obesity (n = 551) | P Valueb |
---|---|---|---|---|
Infancy | ||||
Birthweight, mean (SD), kg | 3.6 (0.4) | 3.6 (0.4) | 3.5 (0.4) | .67 |
Male, % | 52.9 | 59.0 | 51.5 | .13 |
Breastfeed ≥6 months, % | 63.4 | 63.6 | 63.3 | .96 |
Iron status during infancy, % | ||||
Iron sufficient | 41.5 | 34.4 | 43.0 | .06 |
Iron deficient | 40.1 | 40.2 | 40.1 | |
Iron deficiency anemia | 18.4 | 25.4 | 16.9 | |
Socioeconomic status, Graffar indexc, mean (SD) | 27.0 (6.3) | 27.0 (6.2) | 27.0 (6.3) | .87 |
HOME scored, mean (SD) | 30.2 (4.7) | 30.2 (4.8) | 30.2 (4.7) | .90 |
Supplementation group, % | ||||
High iron | 47.2 | 45.9 | 47.6 | .96 |
Low iron | 2.7 | 3.3 | 2.5 | |
No added iron | 42.1 | 42.6 | 41.9 | |
Neuromaturation studye | 8.0 | 8.2 | 8.0 | |
Adolescence | ||||
Age at menarchef, mean (SD), y | 12.5 (1.4) | 12.0 (1.4) | 12.5 (1.4) | .01 |
Age at adolescent measurement, y | 16.8 (0.3) | 16.8 (0.3) | 16.8 (0.3) | .48 |
Physical activity scoreg, mean (SD) | 4.1 (1.6) | 4.0 (1.5) | 4.1 (1.7) | .38 |
Obesity at 16 yh, % | 14.1 | 50.0 | 6.2 | <.001 |
Abbreviations: SD, standard deviation; HOME, home observation for measurement of the environment.
a Early onset obesity defined as obese at 5 years of age following World Health Organization z score cut-off ≥2 SD body mass index (kg/m2).
bP value for χ2 test for categorical variables and t test for continuous variables.
c Graffar index is a social stratification tool used to assess socioeconomic status (range 13-78); the higher the Graffar index, the lower the socioeconomic status (21).
d HOME score (range 0-45) is a home environment quality assessment tool; the higher the HOME score, the better the home environment for child development (22).
e Participants in the neuromaturation study were infants found to have iron-deficiency anemia at age 6 months, and the next nonanemic infant (control) whose neurodevelopment was evaluated with neurophysiological and electrophysiological techniques (20).
f Female participants only.
g Physical activity score (range 0-10) assessing planned and unplanned physical and sedentary activities (26).
h Obesity at 16 years defined as obese at 16-year follow-up according to the World Health Organization z score cut-off ≥2 SD for body mass index.
Table 2. Metabolic Syndrome Risk Scorea and Cardiovascular Disease Risk Factors Among Participants (N = 673), Study of Relationship Between Early Onset Obesityb and Risk of Metabolic Syndrome Among Adolescents, Santiago, Chile 1991–1996 and 2009–2012c
Variable | Total Population (N = 673) | Early Onset Obesity (n = 122) | No Early Onset Obesity (n = 551) | P Valued |
---|---|---|---|---|
Metabolic syndrome risk z score | 0.3 (0.8) | 1.0 (0.8) | 0.2 (0.8) | <.001 |
Waist circumference, cm | 81.3 (11.4) | 94.0 (12.5) | 78.5 (9.0) | <.001 |
HDL cholesterol, mg/dL | 40.2 (10.6) | 37.1 (9.5) | 40.9 (10.7) | <.001 |
Triglycerides, mg/dL | 88.2 (50.0) | 103.3 (57.2) | 84.8 (47.7) | .001 |
Systolic blood pressure, mm Hg | 111.7 (10.5) | 117.5 (11.7) | 110.4 (9.8) | <.001 |
Diastolic blood pressure, mm Hg | 69.1 (7.1) | 71.9 (7.2) | 68.5 (6.9) | <.001 |
Fasting blood glucose, mg/dL | 88.6 (9.5) | 89.5 (12.2) | 88.5 (8.8) | .36 |
Abbreviations: HDL, high-density lipoprotein.
a Metabolic syndrome risk z score calculated with sex-specific and race-specific equations with confirmatory factor analysis.
b Early onset obesity defined as obese at age 5 years according to World Health Organization z score cut-off ≥2 standard deviations for body mass index (kg/m2).
c Values are unadjusted mean (standard deviation) unless otherwise indicated.
dP values calculated with t test for continuous variables for differences between the early onset obesity group and no early onset obesity group.
Table 3. Linear Regression Models to Determine Adjusted Associations With Participants’ (N = 673) Metabolic Syndrome Risk Scorea, Study of Relationship Between Early Onset Obesity and Risk of Metabolic Syndrome Among Adolescents, Santiago, Chile 2009–2012
Variable | Full Modelb | Final Modelb | ||
---|---|---|---|---|
β (95% CI) | P Valuec | β (95% CI) | P Valuec | |
Early onset obesityd | 0.29 (0.15 to 0.44) | <.001 | 0.27 (0.13 to 0.41) | <.001 |
Obesity at 16 ye | 1.18 (1.02 to 1.34) | <.001 | 1.20 (1.04 to 1.35) | <.001 |
Femalef | −0.29 (−0.40 to −0.18) | <.001 | −0.26 (−0.35 to −0.17) | <.001 |
Birthweight, kg | 0.08 (−0.05 to 0.21) | .23 | — | — |
Breastfed ≥6 months | 0.01 (−0.09 to 0.11) | .83 | — | — |
Iron deficient during infancyg | −0.02 (−0.12 to 0.08) | .73 | — | — |
Iron supplementationh | 0.05 (−0.05 to 0.15) | .35 | — | — |
Graffar indexi | 0.004 (−0.01 to 0.01) | .32 | — | — |
HOME scorej | 0.003 (−0.01 to 0.01) | .54 | — | — |
Age at menarchek | −0.01 (−0.06 to 0.05) | .83 | — | — |
Age at adolescent measurement | −0.02 (−0.20 to 0.18) | .86 | — | — |
Physical activity scorel | −0.03 (−0.06 to 0.01) | .10 | — | — |
Abbreviations: —, not calculated for parsimony; CI, confidence interval; HOME, home observation for measurement of the environment.
a Metabolic syndrome risk score calculated with sex-specific and race-specific equations with confirmatory factor analysis.
b Linear regression modeling, presenting β estimate and 95% confidence interval.
cP values calculated by linear regression modeling, adjusted for all other listed variables in each of the models (full and final).
d Early onset obesity defined as obese at age 5 years according to World Health Organization z score cut-off ≥2 standard deviations for body mass index (kg/m2). Reference: no early onset obesity.
e Obesity at age 16 years defined as obese at 16-year follow-up according to World Health Organization z score cut-off ≥2 standard deviations for body mass index. Reference: no obesity at age 16 years.
f Reference: male.
g Includes iron deficient and iron deficiency anemia. Reference: iron sufficient.
h Iron supplementation includes: high-iron and low-iron supplementation during trial. Reference: no iron supplementation.
i Graffar index is a social stratification tool used to assess socioeconomic status (range 13-78); the higher the Graffar index, the lower the socioeconomic status (21).
j HOME score (range 0-45) is a home environment quality assessment tool; the higher the HOME score, the better the home environment for child development (22).
k Age at menarche for female participants.
l Physical activity score (range 0-10) assessing planned and unplanned physical and sedentary activities (26).
The opinions expressed by authors contributing to this journal do not necessarily reflect the opinions of the U.S. Department of Health and Human Services, the Public Health Service, the Centers for Disease Control and Prevention, or the authors' affiliated institutions.
- Page last reviewed: October 12, 2017
- Page last updated: October 12, 2017
- Content source:
- Maintained By: